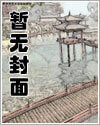
爱嫉妒的女神与孤独的王
作者:冬天看荷花爱嫉妒的女神与孤独的王最新章节:
第274章:找个女人勾引他更新时间:2024-04-27 05:05
邵慕辰摸了摸她的脑袋,“行,我先再看看,做个大概的选择,最后再让你自己决定。”
凌晨曦嗔了一眼邵慕辰,嘟嘟嘴,“慕辰哥哥,我就是想要了解下你公司。当然我也问了下你个人的情况,我这是关心你那。”
厉父的话被厉母打断,不满的拍了下他,“别乌鸦嘴。你该祈祷我们儿子恋爱顺利,早点结婚啊!”
推荐阅读: 美女的透视神医、 碧血丹心逍遥剑、 情深不负,总裁好久不见、 主神多如狗、 末世武学系统、 我就水井有点怪、 变身少女的星辰大海、 调皮王妃别逃、 神河初始、 借酒撞江湖、 超神之幻想系统、 神宠又给我开挂了、 修士的厨神生活、 死后结婚包分配、 虚伪王权、 窗外是你、 雍正皇后生存录、 鱼和刘辉、 ,我的赛博朋克编年史、 双生帝少虐妻成瘾、 九玄太上经、 活了十七年才知是全息